hls4ml
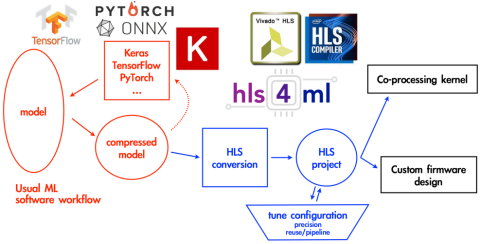
hls4ml is one of a number of environments that support behavioural design for machine learning. It is a Python package that takes machine learning models and translates them into an High Level Syntesis (HLS) implementation. The resultant HLS implementation is used to produce IP which can be made part of an SoC design or used to create a kernel for CPU co-processing. hls4ml has various configuration options to balance model performance, hardware resource utilization and system latency in the way the model is translated into HLS. It allows behavioural design prototyping by iteratively adjusting the configuration, generating the HLS implementation and then evaluating the performance of the system.
Network compression is a widespread technique to reduce the size, energy consumption, and overtraining of machine learning models. Like many alternative environments hls4ml adjusts the precision of input model to a reduced form to better fit hardware resources and the reduce latency and energy cost of execution with some loss of model performance. It has profiling tools to assist in deciding how precision is reduced. To establish whether a configuration gives reasonable model performance C Simulations are executed with test data and compared with results for the model evaluated on a CPU with the original precision.
Configuration of a resource “reuse factor” parameter adjusts a balance of hardware resource use, degree of parallelism and latency. It determines how often resources are reused in order to compute a layers output values. The more resources, the more parallelism, the lower the latency.
hls4ml runs a sequence of general and custom Optimizer passes over an evolving internal model graph. New optimizers can be registered with the environment to add additional translations to the hls4ml conversion process. optimizers are ordered in a flow until they no longer make changes to the model graph.
Like many model translation environments, hls4ml is expanding to cover more input ML frameworks, model architectures and HLS backends.
The HLS backend, 'VivadoAccelerator Backend', utilises the PYNQ software stack to deploy models on PYNQ supported FPGA devices. hls4ml creates via a Python API an overlay for the translated model that can be instantiated, via a bit file, in programmable logic (PL) circuits of the FPGA. The bit file for overlay, which contains the model IP and logic to send and receive data via AXI interfaces, is generated by the VivadoAccelerator backend. A config and Python driver for execution on the ARM-based processing system (PS) within the FPGA are generated as well as definition of the input and output data to allocate the buffers for the data transfer between the PS and PL parts of the FPGA. All is loaded into the PYNQ supported FPGA device to enable execution of the model.
To move to an ASIC implementation requires replacement of the hard IP core ARM-based processing system (PS) and the PYNQ environment with actual synthesizable Arm IP and the technology dependent memory for the SoC such that it can boot correctly once the ASIC die is fabricated and communication with an external test board environment.
The approach taken by SoC Labs to design prototyping in FPGA is to instantiate the entire SoC as synthesizable IP within the programmable logic (PL) circuits and connect the various communications channels and GPIO using a test socket instantiated in the FPGA. The PS communicates with the test socket to simulate the test board.
A SoC Labs project looking to use hls4ml will need to rework the interface of the model IP and logic that sends and receives data via AXI interfaces to connect to the bus of the SoC Labs reference design. The Python driver will need to be replaced by software to run on the Arm CPU of the SoC Labs reference design.
An alternate option would be to create an HLS backend for generation of the IP necessary the instantiate the SoC Labs reference design and the model IP.
In this interesting Siemens EDA presentation on Catapult + HLS4ML for Inference at the Edge it is clearly shown why generating the right intermediate code is important to allow efficient ASIC implementation.
David Burnette in this presentation illustrates a hardware design pattern, Sliding Window, and how generating the appropriate code from the HLS4ML environment allows the design pattern to be used to make an efficient implementation.
Comments
Hyperbolic tangent and sigmoid functions
Long Short-Term Memory (LSTM) models can utilise the two activation functions, hyperbolic tangent and sigmoid. The implementation of these functions is important in terms of the use of limited hardware resources, achieving high-precision and speed of execution.
HLS4ML allows evaluation of such functions in two ways, using a standard math library or via a user defined approximation lookup table.
With a look up table the function is approximated by a fixed number of points on the function. It provides a fast speed of execution of the function involving only a single memory-access to produce a result. There is a trade off between precision and use of limited hardware resources, in this case, the amount of memory needed to store the look up table.
In this paper on Sigmoid Function Implementation quotes a degree of accuracy deviation ranging from -0.005 to 0.005 for 16 Kb of memory and goes on to propose other implementations with more efficient hardware implementations. In this paper on Approximating Activation Functions considered the error in such approximations in activation functions in neural networks. There are a number of papers on this topic.
Another HLS4ML resource on hyperbolic tangent and sigmoid
I found another resource on HLS4ML implementation of hyperbolic tangent and sigmoid functions considering the required fixed point precision and the size of look up table with an implementation now available in hls4ml.
Add new comment
To post a comment on this article, please log in to your account. New users can create an account.