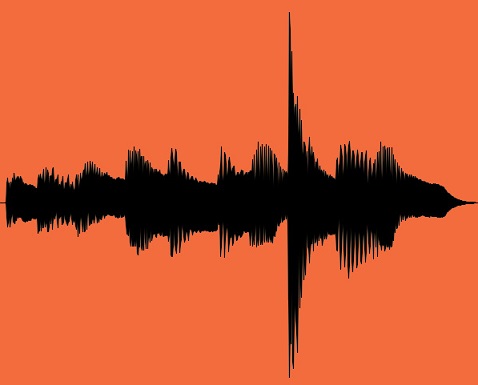
The dynamic range processor is a DSP function which does as it says on the tin; it compresses the dynamic range of the incoming signal. This is used most commonly in the music industry for its effects on the perceived loudness of audio. It is also used extensively in hearing aids to compensate for the user’s reduced dynamic range of hearing. In this project a hardware accelerator is developed for the purpose of dynamic range compression of digital audio. This accelerator will be implemented in a mixed-signal infrastructure.